The global cooperation realized in connection with the COVID-19 epidemic exemplifies how the genetic data of a large number of known clinical individuals can explain the individual differences in the course of the disease. Large data sets can predict the genetic constellation that encodes an endangered condition, they can be the basis of a data-driven strategy for public health programs, and they can also identify targets for drug development. The strategic importance of health data supplemented with genomic data was recognized early on by several countries (e.g. the Estonian biobank or the Finnish Biobank – FinnGen, where genomic data is combined with health data from the national health register). The uniformly managed databases of biobanks are an important part of clinical and translational research, in making personalized therapy and evidence/data-based decisions. This data is extremely sensitive and therefore cannot be shared in the traditional way.
One of the main efforts of biomedical consortia is the cross-border collection and sharing of large amounts of health data consisting of different data types. An important obstacle to data sharing is the lack of trust in data collectors/processors on an individual level, which is why data protection guarantees are needed. Even at the institutional level, individual data processors follow different practices, despite the fact that their goal is to create collaborative models that result in mutual benefit. The problem with international data sharing is similar, it often means a larger scale in terms of element numbers only.
The biggest challenge in exploiting the data wealth created in the healthcare sector to make it manageable is the following,
- it is missing or not common standard terminology and ontology in some data domains;
- lack of access to the necessary technologies/computing infrastructure,
- issues of data protection and data security
- due to the vulnerability of data warehouses and cyber infrastructure to attacks, the centralized storage of data poses a higher risk.
- security risks of Big Data: 1) unauthorized access to data and analysis technologies 2) use of Big Data technologies developed to integrate existing data for the purpose of designing a harmful biological agent.
Our client’s Biobank Network keeps the data of approx. 100,000 individuals. This data asset is managed by diverse, fragmented, non-interoperable data collection systems. The safe sharing of sensitive health data has not yet been developed in Hungary. The prerequisite for international connection is to ensure GDPR-compatible data harmonization within the institution, the sharing of sensitive data in such a way that they cannot fall into unauthorized hands, but while ensuring the maximum protection of personal data, they can still be exploited for the R+D+I area. The data repository unifying the databases of the client will be the beginning of not only biomedical research, but also a wealth of data showing an unbiased picture of the health status of Hungarian society, with the help of which we can make estimates of the nation’s future health challenges.
Solution
The analysis of comprehensive genomic and clinical data based on a large number of samples has a great impact not only on the health of the individual, but also on society. The knowledge and technology developed during the implementation of our pilot project supports the implementation of national and international projects with a similar theme. The client’s immediate goal is to create a network of large-scale federated data sources standardized to a unified data model, which creates the basis for the sharing of national genetic data ensuring data protection. The characteristics of the developed system are: (1) transparency, which enables the availability of the sample found in the biobank network and the inclusion of related data in anonymous shared analyses, (2) interoperability, which lays the foundations for integration into the institutional-national-international network, (3) federated data sharing, which ensures the sharing of sensitive data elements without risks. In order to implement the above, it is necessary to harmonize the data stored in SE biobanks and individual domestic institutions, and to develop a unified data model.
Proposed system
In the project we create interoperability between fragmented databases at the institutional level and to make it possible to jointly analyze them in a way that guarantees the security of the individual’s personal data. In this way, we create a unique cooperation model for R+D+I initiatives. We create federated data assets at the institutional level that preserves data protection guarantees, which supports interactive cooperation between cross-border health data repositories, the identification of new knowledge, a better understanding of the pathomechanism of disease, with the support of clinical research and decision-making. Data sharing that ensures privacy will ensure optimal interoperability between data collection centers.
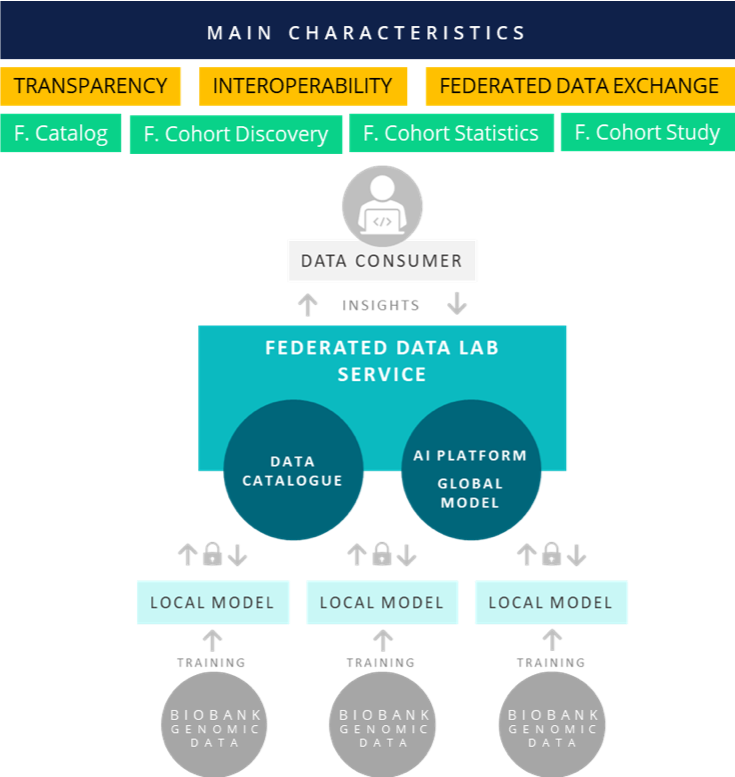
The implemented platform the following tasks must be performed:
Future prospects, scalability of the solution
- harmonized biobank databases: institutional uniform minimally expected data set is developed, coded with common hierarchical ontologies,
- secure, distributed execution environment: development of a system in which algorithms based on artificial intelligence (AI) access sensitive data at their source, only the learned model is shared between the parties during the federated learning process. In addition to model-level masking, additional protocols that preserve security and personal data sensitivity (e.g. private communication channels, secure aggregation, differential privacy) ensure the protection of the system from external attacks, full masking of personal data and their untraceability. During the project, certificates supporting the appropriate security level is being obtained.
- analytical and virtual data exchange system: we are developing a system based on federated learning, which ensures that the data of the biobanks integrated in the project can be uniformly analyzed without the actual personal data being accessed by anyone other than the managing institution. The system enables federated, privacy-preserving access to separately managed sensitive data for running machine learning algorithms or aggregated cohort statistics. Functions of the system includes:
- Federated Cohort Discovery
- Cohort patient counts
- Federated Biobank Catalog
- Secure partner search function based on cohort counts
- Federated Cohort Statistics
- Aggregated cohort phenotype statistics
- Aggregated cohort genotype statistics (e.g. frequency of one or several specific variants (rare mutation or common polymorphism))
- Federated Cohort Study
- Federated genetic data analysis tools (e.g. association between one or several genetic variants and phenotype character(s) in the studied subgroup (PheWAS))
- Federated Cohort Discovery
The federated data network created by the FedX federated learning platform creates the foundations of a national genomic data lake, which, in communication with the national health system, would raise Hungary to a prominent place in the international field, similar to Estonia, in terms of the implementation of precision medicine. Our client can play an important role in joining the 1 Million Genomes program, as it is currently one of the institutions where most complete genome/exome sequences are available. Our long-term plan is also to develop safe methodology and know-how for the emerging European Health Data Space data service program, including the TEHDAS (Joint Action Towards the European Health Data Space) project.